Thus, you are considering implementing generative AI into your enterprise? That’s a fantastic move!
So, even if you plan to immerse yourself in the age of wonders created by artificial intelligence, it is essential to address the data management issue first.
Why? Since, as the proverb goes, you cannot make a silk purse out of a sow’s ear, masterful AI too requires masterful, that is, high-quality data to feed on to be able to generate meaningful results.
Now let’s take a step-by-step on how to develop a data strategy for generative AI that will help you succeed.
Understand Your Goals
So the first question is, what is your objective with generative AI anyhow? Do you need to generate articles automatically, improve customer engagement, create product content, or maybe create iconic designs?
Therefore, it becomes essential to give clear goals to define what type of data is required for the evaluation of your business. As we can see, it is much easier to make the right decision when you are sure of the right direction that you want to take, even when doing a road trip and setting your destination is all that you need.
We are not talking about vagueness and mere awareness of the goals; it calls for goals that you can quantify. For example, if you want to automate content creation, you should determine what types of content are needed and in what amounts: blogs, posts on social networks, descriptions of products, etc. It assists in defining the quantum and nature of the data needed.
Besides, goals and objectives will assist in establishing the degree of success in the generative AI technology initiatives, known as KPIs.
Do you want to cut the time for content creation by half? Or perhaps you want to lift the click-through rate of social ads by 30 percent? These specific targets will help in focusing on data and ensure that AI initiatives are aligned with the organization’s goals.
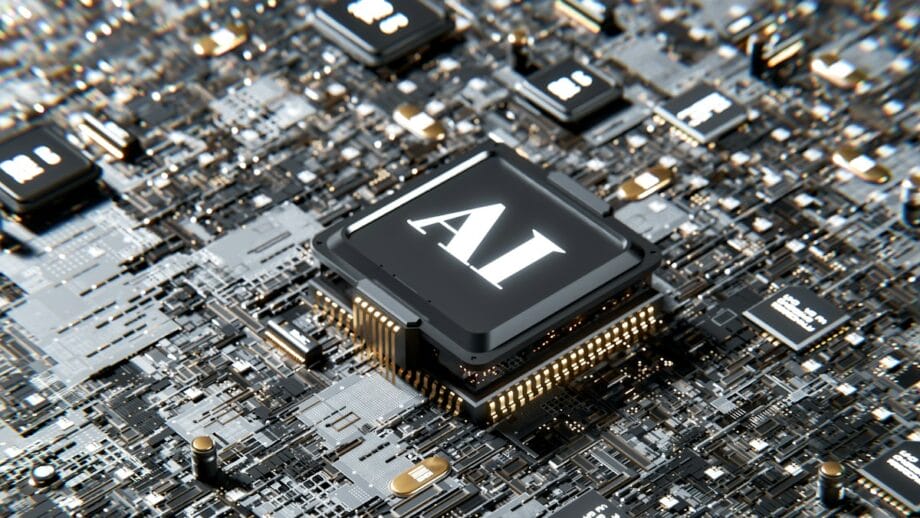
Identify the Right Data
Finally, when the goals are identified, the next step is to define exactly what data is required. Generative AI depends on data, and the more extensive and varied that data is, the better it performs.
As for text creation, the creation of any necessary texts will require an enormous database of good-quality written material. As for image generation, the heterogeneity of images is vital. It is, of course, akin to having all the ingredients one could need to make any meal they want. Ensure that your data is accurate, current, and, above all, reflects realistic outcomes.
Identifying the right data involves understanding the sources and types of data available. For text generation, consider a mix of structured data (like databases) and unstructured data (like articles, reports, and social media content). For image generation, your dataset should include various styles, genres, and contexts to ensure versatility in the AI’s outputs.
Additionally, consider the legal and ethical implications of your data sources. Ensure that the data is obtained legally and respects intellectual property rights and privacy laws.
Data Quality is Key
It is one thing to have the right data and quite another to guarantee that the data is clean. Since the models of generative AI are based on data, they are as good as the data used in the training process. This is what you get: garbage in, garbage out—this is the philosophy of the company.
Where data is clean, well organized, and adequately annotated, the AI and accordingly the output will also be better and more accurate. It is just like adding new authentic and premium-quality ingredients to your cooking, and the outcome is much more delicious.
To ensure the data quality that will be used in the analysis, it is advisable to follow the proper data-cleaning procedure. This entails handling the data to clean it such that duplicates are eliminated and formatting errors corrected.
Moreover, it is important to do proper annotations of the data. In the case of text data, this may mean things like POS tagging or sentiment analysis, while the same for images could be object/scene tagging.
To prevent the decline in the quality of your data over time, it would be useful to do periodic data audits. Use automated forms and frequent self-verifications to keep your data clean and up-to-date.
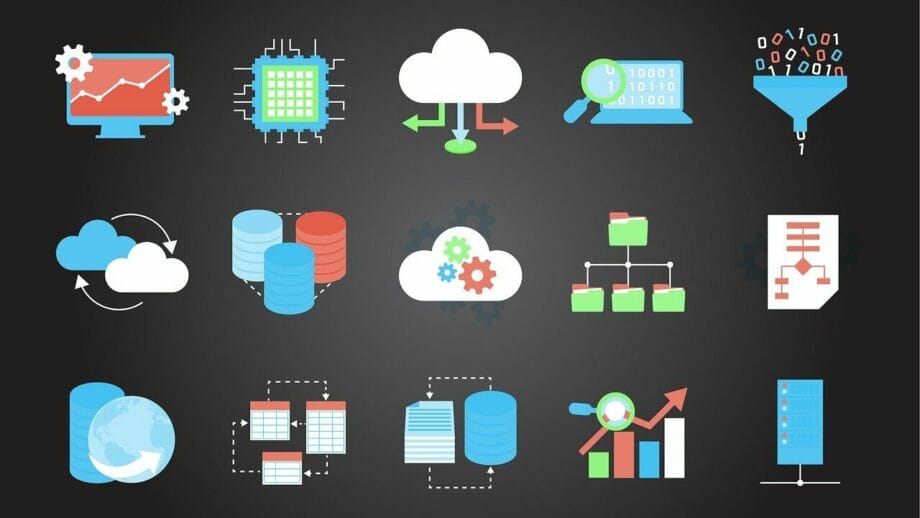
Establish Data Governance
Data governance does not sound very exciting, but it is critical. With regards to the management of the data, entails implementing some measures or policies that would enable one to manage the data correctly.
This particular step may be compared to the assistant director, who oversees the activities in the set to ensure no interferences, thus allowing the director to direct. Implement roles and responsibilities for the management and achieve compliance with data and data standards as well as regulations.
The first principle of data governance focuses on role clarification concerning different data management activities. This would consist of data stewards, the data custodians, as well as the data users.
Data stewards have holistic responsibility for data, data custodians are technically responsible for the data, and the data users utilize the data correctly. Formulate and communicate explicit guidelines dealing with data quality, data security, data confidentiality, and the usage of data in the organization’s various phases. One framework that can be adopted is the DAMA-DMBOK Data Management Body of Knowledge.
Ensure Data Security and Privacy
As with everything in life, access to a lot of data requires the corresponding level of sensitivity and the ability to analyze and apply such data. Security and privacy of your data are of the utmost importance. Ensure you have adequate security policies that will check the leakage or theft of your information.
Sarbanes-Oxley Act 2002 and California Business and Professions Code Section 22580 to respect users’s data and privacy, GDPR, CCPA, etc. I suppose it can be compared to the way people protect the key to their pantry and therefore the ingredients.
That is why data protection also implies the use of physical and digital security measures. In this respect, it entails the physical security of servers and data centers.
Technologically, it includes encrypting data and passwords and regularly conducting security assessments and authorizations on the data. Yet, have a well-articulated data breach response plan through which you can respond to any mishap at the earliest.
Privacy is equally important. Make sure that you are gathering the minimum amount of information that will suit your purpose, and if you intend to ask users for permission to use their information, then make sure that you state it clearly. Ensure that your privacy policies are written and up-to-date to conform to the present trends in the market.
Use the Right Tools and Technologies
Finding the right instruments and tools for organizing and further processing your data is rather important. Provide data lakes, data warehouses, and AI platforms that are scalable enough to process all incoming data. These services, such as Azure, AWS, and Google Cloud, have capable solutions for MCS storage, MCP processing, and MCAnalysis.
It is a situation similar to being given brand new, multi-functional kitchen utensils that help in the cooking process in a better way.

In choosing the tools, employing characteristics such as versatility, incorporability, and cost will be key. Currently, data lakes help you store raw data without altering its structure, which can be convenient for future use.
While data marts are the repositories of raw data that has been collected and fed to the organization and might be unstructured in some cases, data warehouses contain clean data that have gone through processing and structuring to be queried and used for reporting purposes.
AI platforms are custom-built systems that provide ready-made templates and frameworks that can help in the development as well as the implementation of AI solutions. Assess one vendor against another depending on the features of services they offer, their support systems, and customers’ real-life testimonies.
Continuous Improvement and Monitoring
It is also crazy to have a data strategy that does not evolve. Regularly review and update it by developing new skills and knowledge about the company and industry. Take the steps to examine the performance of your generative AI models, and if any improvements should be made, then make these.
Just as you manage your food and keep sampling it to get the best taste, that is how the market operates with continuous sampling of the market environment. Ask for and collect opinions and reviews on the outcomes of your AI, and also strive to improve your method to get the best outcomes constantly.
Thanks to incorporating the idea of continuous improvement, one must establish a feedback system that would allow for evaluating AI experience, identifying any potential deviations from the desired outcome, and making corporations if necessary. The assessment of the models must be done using accurate criteria such as accuracy, relevance, and user satisfaction.
Use A/B testing to cast versions of the outputs of your AI against each other. Always update the models with new data so that the results are quite accurate in addressing the issues. Ensure to closely follow the advancements in the use of AI and data management to utilize all the best practices there are.
Foster a Data-Driven Culture
Lastly, incorporate a culture that supports the proper use of data in your organization. Make it a management best practice that exudes your team to engage the power of data insights and to look for opportunities on how the organization can get more value from it. Calm and efficiency of a peaceful kitchen where all the people welcome the high-quality products and good cooking.
Yes, data culture is a top-down process, which means that it needs to be introduced at the managerial level. The use of data to make decisions should be promoted by the leadership, and proper resources should be assigned to support data projects.
Educate workers; equip them with knowledge and materials that would aid in enhancing relative data skill sets. By doing this, one will be in a good position to guide his subordinates, supplement their successes through celebrations, and learn from their failures to make steady progress in building a positive culture.
Promote cross-functional communication to share the analytics results and progress with the initiative as a single team.
Conclusion
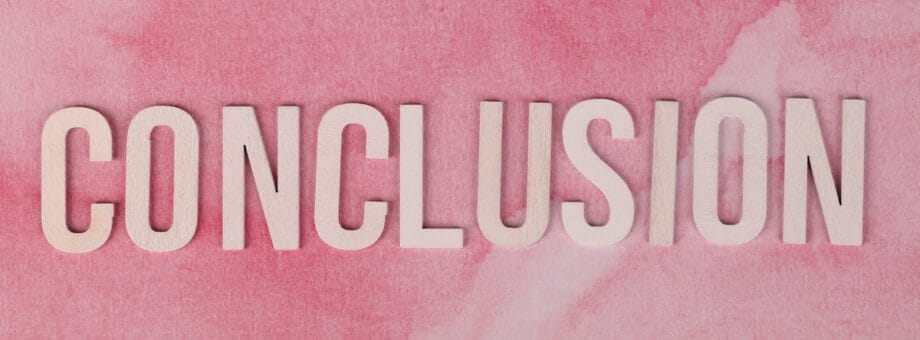
Integrating generative AI into your business can be a game-changer, but it requires a solid data strategy. By understanding your goals, identifying and ensuring data quality, establishing data governance, securing your data, using the right tools, continuously improving, and fostering a data-driven culture, you’ll set the stage for AI success.
Remember, your AI is only as good as the data it’s fed, so treat your data strategy as the secret recipe to your AI’s success. Ready to get started? Let’s cook up something amazing with generative AI!
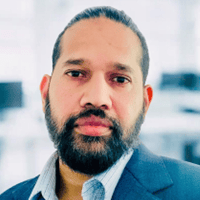