Organizations today depend heavily on data to make decisions that enhance operations and obtain marketplace advantages because of huge data collection volumes. Big data expansion and complex system structures have made power reliability alongside data fidelity become major execution obstacles.
The intersection of crucial data management concepts known as data observability and data quality emerges. Data management strategies become better through integrating these learning fields, enabling companies to defend information integrity with optimal accessibility of operational data.
This publication demonstrates that the combination of data quality with data observability creates new possibilities to optimize business performance against market rivals.
Modern data management systems use a fundamental understanding of both data observability and quality functions.
Understanding Data Observability and Data Quality
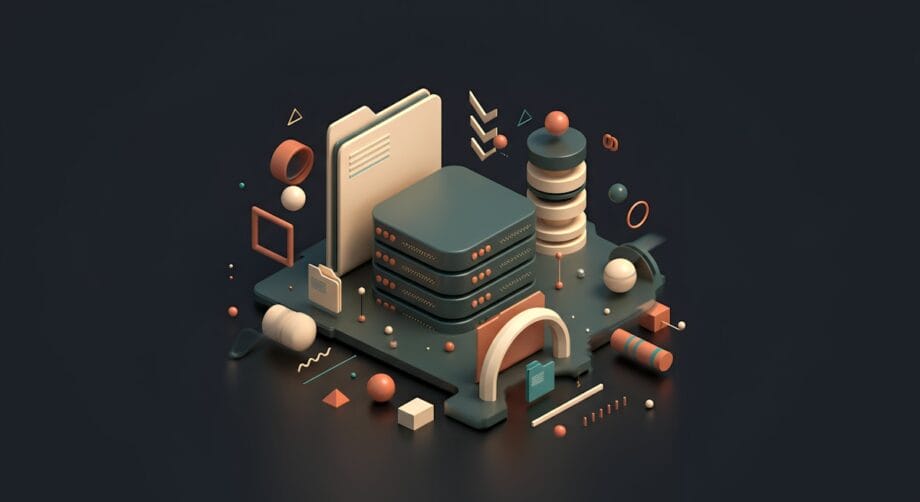
Data Observability joins forces with Data Quality to form the basis of current data management systems. These two concepts need a clear understanding before discussing their combination.
1. Data Observability
Data observability stands as the capability to track data systems in real-time for troubleshooting purposes, along with understanding their operational state. Organizations monitor data movement through systems by using tracking systems to validate smooth pipeline performance.
The implementation of tracking systems leads to dependable data transfers from origin points to target destinations. The system’s current operational state becomes visible to users through operational intelligence, which detects system problems together with outliers while reporting performance delays.
2. Data Quality
The core target of data quality research centers on three areas, which include accuracy, completeness, consistency, and reliability. Purified data contains no mistakes, repeated information, or contradictory elements which produce reliable data for assessment and decision processes. Initiatives around data quality typically include data cleansing, after which comes validation, along with enrichment of available data.
Data-driven organizational excellence now requires tighter integration between previously segregated disciplines so companies can create stable information systems.
Why Merge Data Observability with Data Quality?
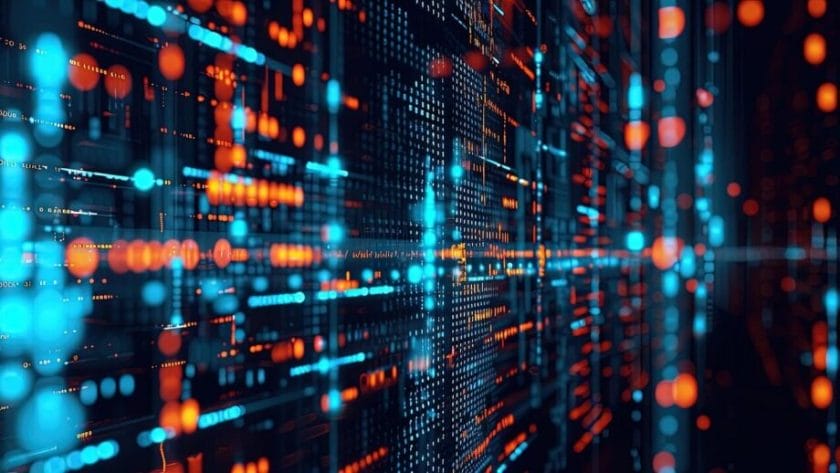
Integrating data observability with data quality is essential due to the growing need for proactive data management in modern organizations. In today’s fast-paced business environments, organizations must identify and resolve data issues before they lead to operational disruptions or impact decision-making. Ensuring accurate, trustworthy, and actionable data requires systems that can detect anomalies and correct them in real-time.
Here’s why this integration matters:
1. Real-Time Issue Detection
Real-time data observability tools provide insights into how data pipelines operate, enabling teams to detect and address issues before they cause operational failures. By combining these tools with data quality metrics, businesses gain immediate awareness of missing information, unexpected schema changes, and unusual value patterns. This ensures that data remains accurate, consistent, and reliable across all processes.
2. Improved Data Trust
For organizations to make confident, data-driven decisions, they must have trust in their data. Merging data observability with predefined quality standards ensures that data flows consistently and reliably throughout systems. This integration reduces errors, enhances data integrity, and builds stakeholder confidence, ultimately minimizing the risks associated with inaccurate or incomplete data.
3. Operational Efficiency
Integrating data observability and data quality simplifies data management by automating monitoring and validation processes. This reduces the time and effort required for manual inspections and troubleshooting, lowering maintenance costs for data systems. As a result, IT and data teams can focus on higher-value business activities instead of constantly fixing data pipeline issues.
By merging data observability with data quality, organizations ensure that their data remains reliable, actionable, and optimized for success in an increasingly data-driven world.
4. Scalability
Organizations cannot sustain traditional methods to manage data quality while data volumes, along with complexity, continue to increase. Through data observability, companies can monitor large datasets along with complex pipelines while maintaining quality standards at any scale.
Key Components of a Unified Data Management Framework
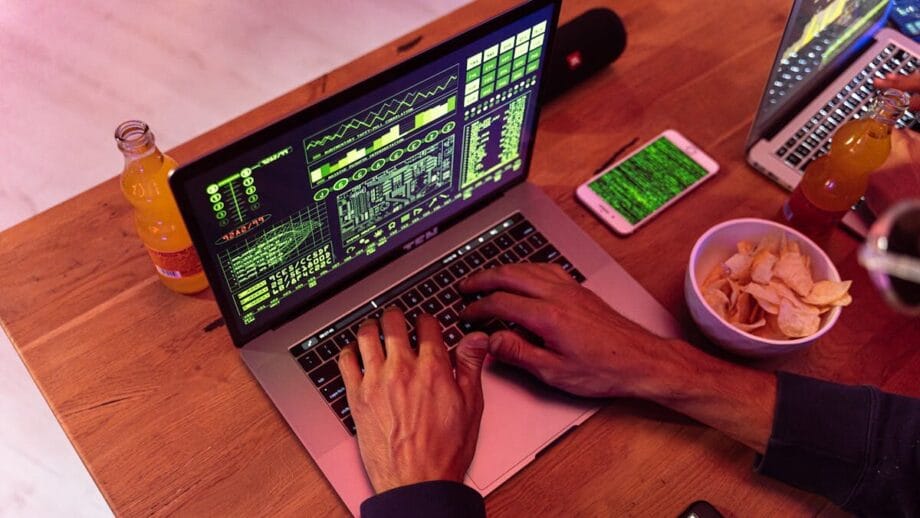
To successfully merge data observability with data quality, organizations need to adopt a unified framework that incorporates the following components:
1. Comprehensive Monitoring
Develop tools for end-to-end visibility of data pipelines including metrics for latency, throughput, and error rates. These tools should also track data quality in terms of accuracy, completeness, and consistency. Some of the best data observability tools in the market include Monte Carlo, Bigeye, and Databand, which offer robust monitoring and alerting capabilities.
2. Automated Alerts and Remediation
Organizations should implement automated notification systems to promptly alert teams about potential data issues, such as data drift events, schema changes, and quality violations. These systems provide real-time insights, allowing teams to respond before significant disruptions occur. Advanced AI-driven remediation tools can also detect anomalies and automatically resolve issues without human intervention, reducing downtime and ensuring data integrity.
3. Data Lineage and Traceability
Data lineage tracking systems allow users to monitor data movement and transformations from source systems through their entire lifecycle. By maintaining visibility into how data is processed and altered, organizations can quickly identify and resolve data quality issues at their origin. Additionally, robust lineage tracking supports regulatory compliance requirements, ensuring organizations meet industry standards and avoid compliance risks.
4. Collaboration and Governance
For effective data management and quality control, organizations must establish clear roles and responsibilities that promote collaboration between data engineers, analysts, and business users. By defining governance policies, companies can ensure that data quality standards are consistently upheld across all departments. A well-structured data governance framework fosters transparency, accountability, and better decision-making.
5. Continuous Improvement
Integrating observability insights with quality metrics enables continuous enhancement of data processing systems. By leveraging machine learning algorithms, organizations can analyze historical data to predict potential issues and proactively address them before they escalate. This predictive approach enhances data trustworthiness and ensures that systems evolve to meet future data challenges efficiently.
Applications Across Industries
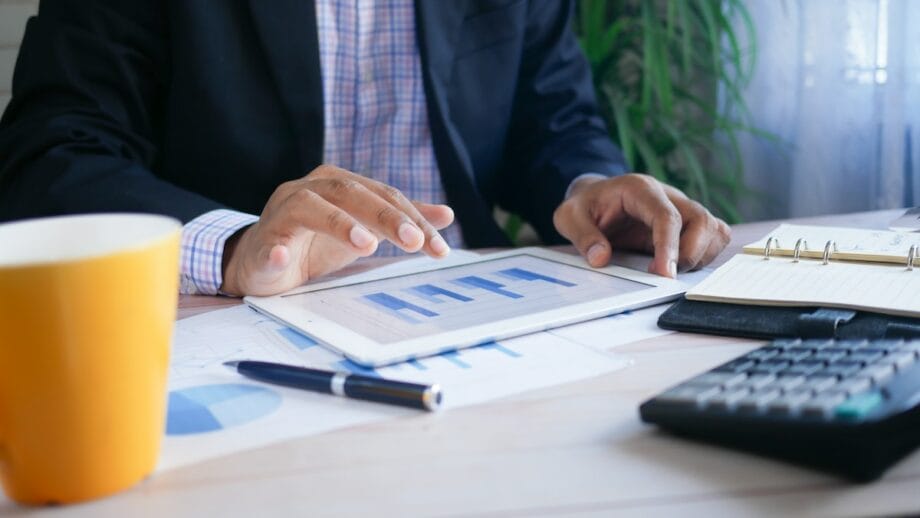
The unification of data observability practices with data quality measures leads to industry-wide improvement in reliable knowledge generation. Here are a few examples:
1. Healthcare
Different healthcare sectors demand complete and timely data since this information is essential both for medical treatment and regulatory requirements. Healthcare providers strengthen patient care through a combined approach of observability and quality management, which ensures real-time accuracy of medical information such as records and treatments.
2. Finance
Financial institutions need correct data for their risk data evaluations, their fraud discovery systems, and their regulatory reporting functions. A single strategic management process for financial information enables both accurate observation and trustworthy use of data, which cuts down on mistakes and regulatory noncompliance.
3. Retail
Companies leverage data to maximize their inventory systems tailor customer encounters and project market requirement forecasts. An integrated observability system combined with quality management enables organizations to use accurate actionable data from sales as well as supply chain and customer feedback sources.
4. Manufacturing
In a manufacturing setting, data derived from sensors and IoT devices is used to monitor the equipment performance and optimize the production processes. A comprehensive data management framework is essential in ensuring a reliable set of data is available, which can be used in predictive maintenance and reduce downtime.
Challenges and Considerations
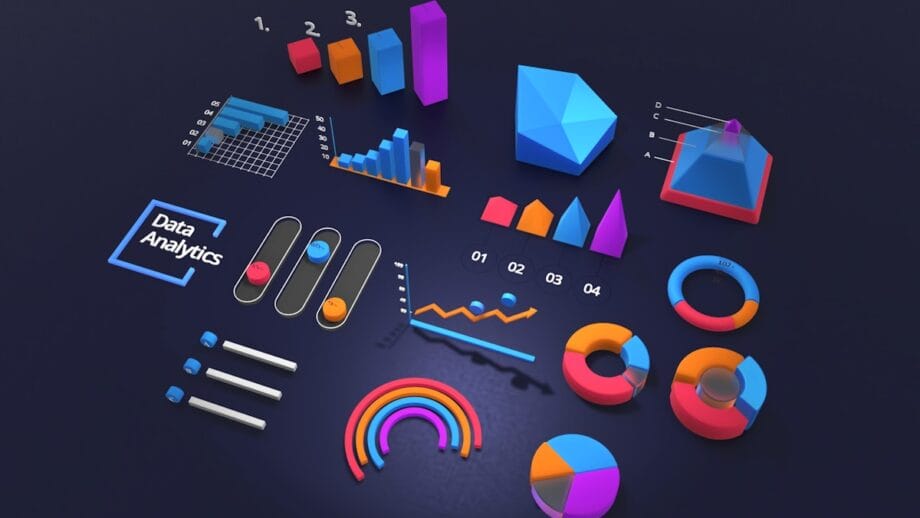
While the benefits of merging data observability with data quality are clear, organizations must address several challenges to ensure successful implementation:
1. Tool Integration
A common organizational practice involves having observability tools in separate systems from quality tools, which generates divided data access and suboptimal operational performance. A uniform information platform enables users to use their data management operations seamlessly.
2. Skill Gaps
A successful implementation of this unified framework demands both observability and quality management expertise. Completely building the required unified framework might demand organizations to provide training programs to their current team members or dedicate a budget to recruiting new personnel who will connect the skill gaps.
3. Cost and Complexity
The effort needed to design and sustain a cohesive data management structure involves substantial costs and complicated development processes. Management teams need to evaluate if the advantages create adequate returns on their financial commitment.
4. Cultural Shift
For successful integration between these fields, organizations should develop proactive cultural shifts that promote data management across teams. The implementation of this change depends heavily on strong leadership support combined with clear communication methods.
The Future of Data Management
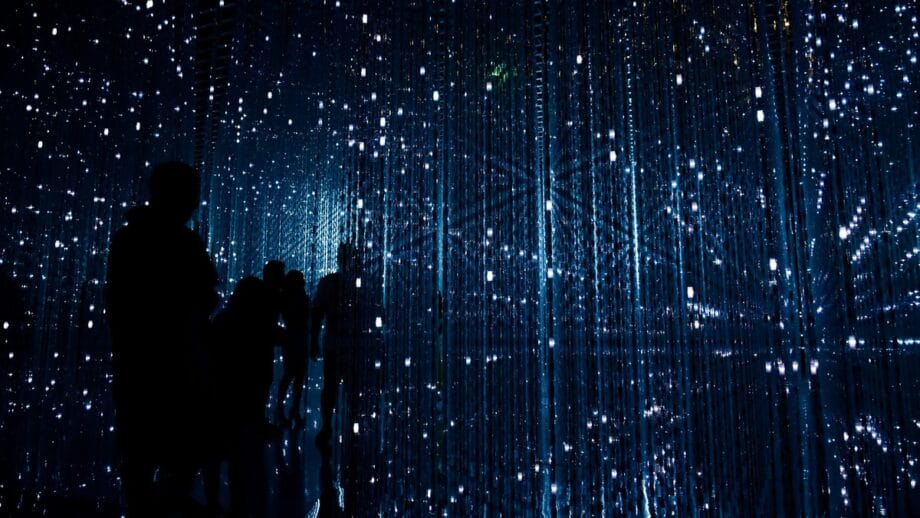
The combination of data observability techniques with data quality solutions demonstrates the direction that data management will take. Real-time data reliability monitoring and maintenance capabilities will establish themselves as critical differentiators that help organizations make strategic decisions based on their data. AI and machine learning developments will boost these capabilities by bringing predictive analytics systems along with robotized resolution mechanisms.
When organizations establish a uniform data management process, they develop trusted data foundations that enable teams to execute quicker decisions supported by solid information. Bosses require the integration of observability and quality beyond best practices to sustain business operations due to data’s vital status as a business lifeblood.
Conclusion
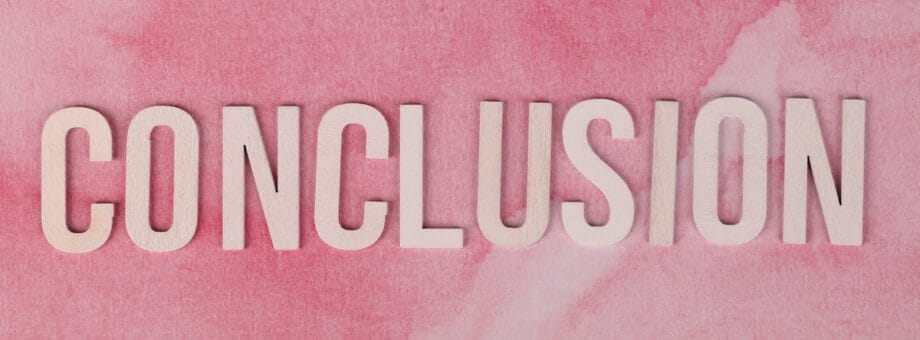
Future data management operates through the unification of data quality processes with data observability systems. These disciplines create fundamental tools and processes that ensure data maintains both accuracy and reliability for actionable use. Organizations that use a unified framework will achieve better results through proactive data issue resolution while earning stakeholder trust and extracting complete value from data resources.
Organizations that unify data observability with data quality operations will successfully face data landscape transformations, which will create enhanced capability to handle complexity alongside innovation-based goal achievement. Data quality requires immediate alignment with data observability tools to build fortified data protection frameworks.