In the current age, data is an indispensable resource to business growth. However, there are several elements and factors involved in maximizing data. All these elements culminate in the concept called data lifecycle.
From collection to usage, data requires quality, diligence, reiteration, security, etc. Furthermore, tracking becomes increasingly complicated as companies get more access to (critical) data. Also, as data volume increases, problems in usage, storage, processing, etc., arise.
Big data then comes into the picture, blowing the issues and complexities out of proportion.
Understanding the data lifecycle’s fundamentals becomes critical to demystify data management complexities. Hence, the need for this ultimate guide to data lifecycle.
What is Data Lifecycle?
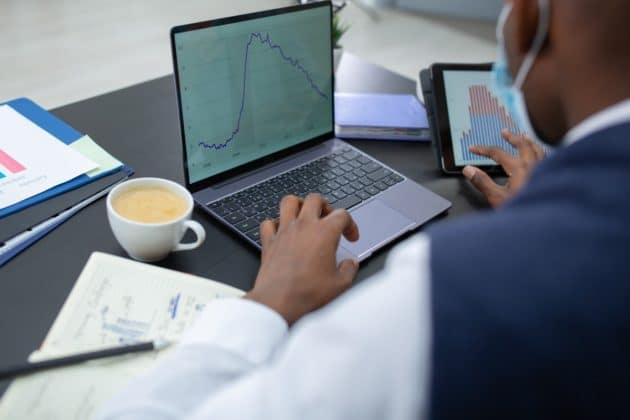
The data lifecycle refers to the total duration a particular dataset spends in your system. As the name implies, a data’s life cycle is all the phases it passes through, from collection to subsequent disposal. The data life cycle doesn’t stop at the end; it’s a loop. The whole process continues to repeat itself from start to finish in a never-ending cycle.
However, not all data sets and types have the same life cycle. Some are churned, and some remain in storage. A major influencing factor for this is relevance. Data perceived to be irrelevant are removed from the database.
In the same vein, valuable data can be improved upon, carefully arranged into classes, or prepared for distribution. These data-related activities culminate in what is known as data lifecycle management.
The complexity of your data lifecycle management depends on the size of your business. As your data architecture becomes more extensive, you may need in-house professionals to manage the systems. In one of the subsequent sections in this piece, we’ll take a detailed look at data lifecycle management.
Recommended for you: Top Tips for Protecting Customer Data.
The Stages of Data Lifecycle
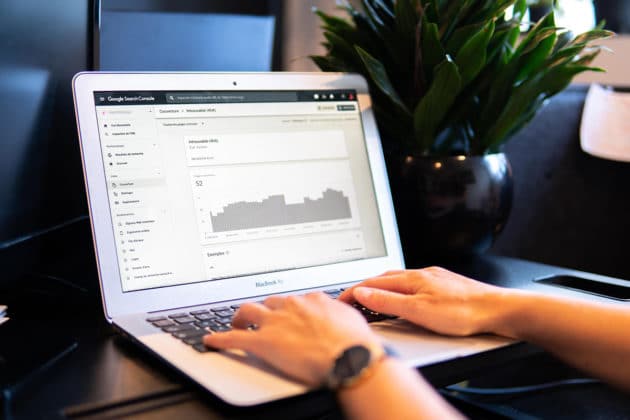
Different institutions have different stages of their data lifecycle. Even within a company, varying data lifecycle can be employed across various departments. Essentially, a company’s data lifecycle depends on its needs and peculiarities. Hence, before executing a data lifecycle, your organization must identify its needs. The data lifecycle employed should have a direct impact on your business.
Fundamentally, a company’s data lifecycle will be premised on the following stages.
Generation
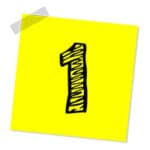
The generation stage marks the beginning of any data lifecycle. Organizations can’t use what they don’t have.
Data generation is so intrinsic to businesses of the 21st century that the process is almost autonomous – nature-like. It can happen even when you aren’t aware of it. Data generation and accumulation have started once your company has social media accounts, a website, or a landing page.
Furthermore, autonomous data generation occurs with your partners, customers, investors, etc. You get data with every sale, communication, hire, interaction, etc. When you pay conscious attention to these details, you’ll notice the dormant data trove.
Collection
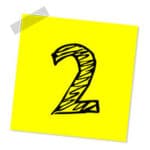
Almost all digital infrastructures today generate data. However, the onus falls on you to collect as much as possible. Though, you can’t collect all. You should set up a system to identify vital data to collect. You must begin churning unnecessary data from this stage.
There are several processes to data collection. These are:
- Forms: With tools like Google forms, Typeform, etc., you can set up data collection pages across your website, social media, etc.
- Surveys: Surveys are a more specific way to generate insights and data from users. They are effective in getting tailored information from many people at once.
- Interviews: Interviews will suffice when you need more in-depth information from your users or customers. Though, this is inefficient if you’re dealing with a large market.
- Direct Observation: This is the most common data collection system. You also don’t need to monitor it every hour manually. Instead, you can observe how users interact with your website or application with automated tools. Having a detailed overview of how users use your platform, what problems they face, etc., helps you improve deliverables.
There are more data collection processes organizations can employ. Identify what works for your company and double down on it.
Processing
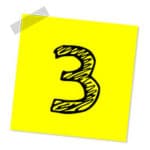
Data becomes useless if it’s not processed. The data processing stage comes in different forms.
- Wrangling: Data wrangling involves cleaning and transforming raw data into more usable formats.
- Compression: Data compression involves converting data sets into more efficient storage formats.
- Encryption: Data encryption translates raw data into encoded characters to protect it.
Storage
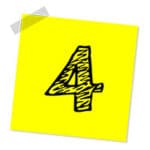
Upon collecting and processing data, it needs to be stored. Data is stored in databases, on-premises servers, or cloud infrastructures. Data can also be stored on minor storage devices like hard drives, cassettes, etc.
Setting up a data storage facility is a complex operation. Hence, ensure it’s carried out by a professional. If yours is a large organization, consider using a cloud data architecture.
Management
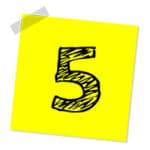
Database management operations include sorting, storing, and retrieving data when needed. The data management process is a massive one, which runs almost perpetually. Every activity that keeps data running from start to finish is a management operation.
Analysis
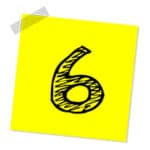
In its raw format, data can be incomprehensible. Analysis helps make sense of it. Data’s purpose is to inform. Without proper analysis, data can’t provide any valuable insight. Data analysts and scientists use different tools and strategies to get the best insight from data.
Some data analysis tools include statistical modeling, artificial intelligence, algorithms, machine learning, data mining, etc.
Visualization
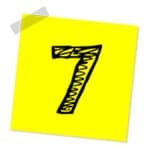
Data visualization involves creating graphical information representations. This data lifecycle stage leverages several visualization tools, which work differently. Visualization helps a company’s management, decision-makers, marketers, etc., better understand the customers.
Visualization, however, isn’t limited to customer-facing data. Any data set can be visualized. The stage is mainly to aid comprehension and understanding.
Interpretation
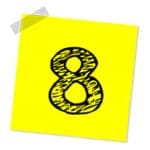
The interpretation phase involves drawing hypotheses and making conclusions from analyzed and visualized data. The quality of data interpretation depends on how well the preceding steps have been executed. Proper data management and analysis lead to accurate interpretation, thereby informing excellent decision-making.
You may like: How Peer-To-Peer Proxy Networks Keep Big Data Honest?
What is Data Lifecycle Management?
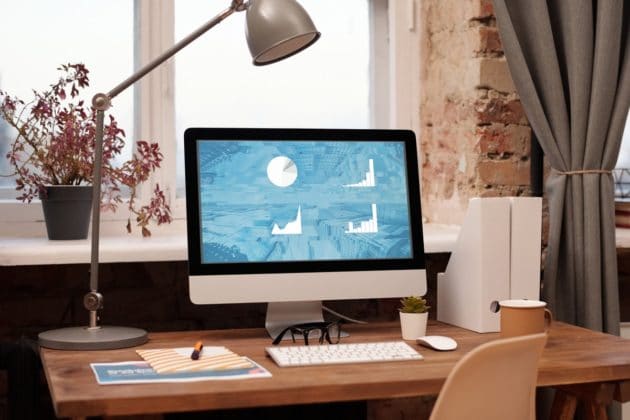
The answer to what is data management lies in the framework. It’s a model that manages data through its entire life cycle. With the data lifecycle management model, data is optimized from collection to deletion. Essentially, the management infrastructure begins with data creation and ends in data destruction or re-use.
The focus of data lifecycle management is optimization.
What are the Main Goals of Data Lifecycle Management?
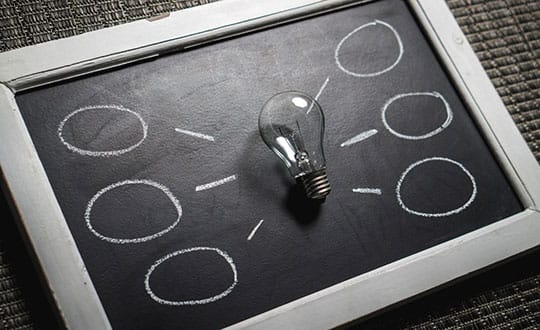
Data lifecycle management is vital to businesses, and it has the following core goals:
Security

Data theft is prevalent in the current world. If a company becomes lackadaisical about protecting its data, breaches and hacks are imminent. However, when the data lifecycle management model is executed optimally, threats and malicious attacks are warded off.
Availability

Data lifecycle management involves preventing unauthorized data access. However, the system also needs to deliver data to the right users at the right time. A data lifecycle management infrastructure that fails at this isn’t optimal.
Furthermore, the data infrastructures should also be capable of delivering the same data to multiple persons simultaneously. Hence, you should pay attention to system bandwidth, load speed, etc. In addition, different teams and personnel in a company may need to pull data at any time. Therefore, it’s pertinent to ensure no impediments to such processes.
Integrity

Data quality is more important than the data itself. It’s not enough to have data; your company must have the correct data. When a company curates, processes and stores unnecessary data, it’s a financial loss.
Also, low-quality data undermines decision-making, marketing strategies, sales pitches, etc. Hence, data quality must be monitored and maintained to standard, right from the collection point.
Furthermore, regular system checks should proceed, aimed at removing outdated data. For example, some data are only relevant for specific periods. It’s wasteful to keep such data around.
How Data Lifecycle Management Helps Businesses
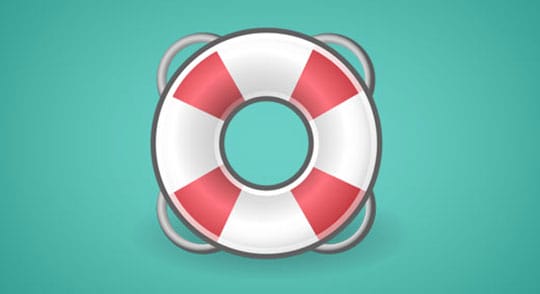
Whether you have a large enterprise, or a small business, access to quality data is game-changing. The following benefits will ensue when you implement data infrastructures tailored to your business.
Compliance to Regulation

Data is available everywhere. However, there are data privacy regulations per industry. With a modern data lifecycle management model, your data processes easily stay within the law’s confines.
Efficiency

Data management can be tasking. But when your business operates a standard data lifecycle management system, the whole data maximization stages become efficient. For small businesses especially, inefficient data systems only waste limited financial resources.
Security

Data lifecycle management prioritizes security. Data that are appropriately secured achieves business goals. You should also set up contingency measures if your security systems get breached. For instance, it’s vital to have an updated backup data system.
Improved data value

Data lifecycle management reiterates every data item, process, and system. Constant data improvement and enrichment improve overall data value. The higher the data quality, the better the decision-making.
You may also like: Top 10 Virtual Data Room Software.
Conclusion
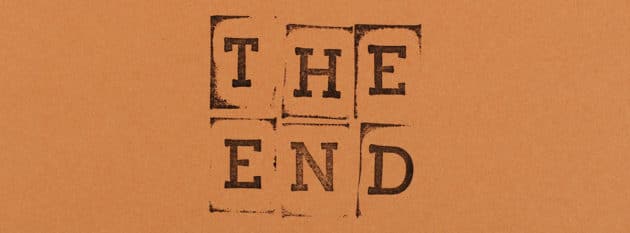
The lifecycle of data is never-ending. Hence, the need for specialized experts who work with every aspect of the framework. Moreover, data has developed to be the heart of every successful business. Hence, it’s important to invest ample resources.
As a business owner or data analyst, it’s essential to prepare your data management systems to scale with your company. As your company grows, big data ensues. Improper and inefficient data management can lead to massive losses.